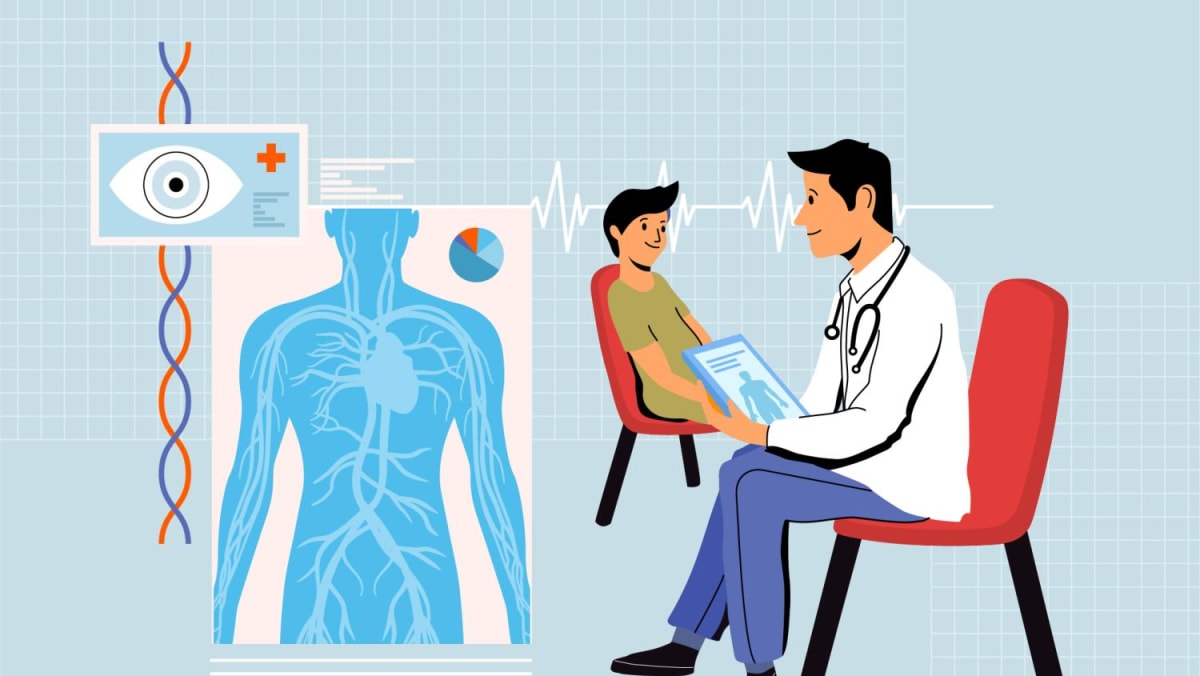
Adrian Yeow
By
Foong Pin Sym
2. Contextualising AI Tools
Another big challenge is making sure AI tools fit well into how healthcare organisations work.
Bringing any new tech into the daily routine of healthcare work requires coordination between experts on the tech, health IT infrastructure teams, and healthcare professionals to make sure the new tools work well in the environment.
Furthermore, it takes a lot of effort to make sure AI tech can work with existing systems, get the right data, and go through the necessary processes, which might involve data stored in different parts of the system.
This is potentially further complicated by the fact that different parts of the organisation have different goals.
On top of dealing with data, the AI tool needs to match the tasks and workflows of healthcare professionals. This might mean changing how things are done and even how the AI results are shown on computers or in the workplace.
Differences in how different people work can cause problems during the integration phase.
3. Enhancing AI Tool Explainability
AI models are often characterised by their inscrutability — they make decisions, but nobody really knows how. This creates a “black box” of decision-making.
The lack of transparency can cause issues when making critical medical decisions.
In a recent AI project at Ng Teng Fong General Hospital, the team, which includes one of the authors, developed a highly reliable Natural Language Processing (NLP) model for predicting sepsis.
However, they hit a roadblock in proving that the important details used in the programme were right. There were variables derived from doctors’ patient notes that made it hard to explain how the programme could predict if a patient might get sepsis.
Moreover, the model’s reliability depended on how well doctors wrote down information. This raised uncertainties about how well it would perform when applied to patient notes from other hospitals.
The problem is, explaining how computer programmes figure things out is so complicated that even doctors struggle to understand.
This puts doctors in a tough spot — they are in charge of decisions made by AI but cannot fully grasp how AI makes those decisions.
This creates an undesirable scenario where the authority of doctors eventually becomes rooted not in their knowledge, but in their role as operators of AI.
Patients then face a dilemma: How do they trust decisions made by doctors using an AI tool if those doctors are unable to fully understand how said tool works?
RECOMMENDATIONS FOR IMPLEMENTING HEALTHCARE AI
Here are three key recommendations for healthcare organisations in introducing AI, focused on three main relationships.
1. AI Developers with AI Evaluation Team
To start, healthcare organisations looking to integrate AI use into day-to-day operations need to form a dedicated, cross-functional AI evaluation team to assess the suitability of new AI tools for such use.
The team should include clinical innovators, data scientists, and medical informatics representatives. The role of team members is to understand and validate the chosen AI model’s performance within the organisation’s specific conditions.
The team’s first task should be to review the AI model’s reported measures, including accuracy metrics and data sources. This review helps explore the model’s core assumptions and relationships.
The next step involves verifying the AI model’s performance using local data and collaborating with clinical experts to cross-check ground truth labels. This process ensures the AI model operates accurately within the organisation’s context.
2. AI Implementation Team with Stakeholders
The second recommendation involves integrating any AI tool into the workflows of target departments. Healthcare organisations should establish an AI integration team, structured like typical enterprise system project teams, including a steering committee, working committee, and AI implementation project teams.
The steering committee, led by senior clinicians and executives, provides leadership and direction for AI implementation. The working committee, led by AI leads, focuses on technical, clinical, and operational integration, addressing privacy, ethics, and safety concerns. AI implementation project teams are responsible for deploying the AI tool and monitoring process metrics, closely coordinating with the working committee to address issues.
3. AI Users and Patients
The final recommendation concentrates on AI users — mainly clinicians and patients directly impacted by AI-enabled healthcare processes.
One strategy is to create interpretable explanations for AI predictions using related but more easily explainable models. Additionally, allowing clinicians and users to query the conditions under which the AI model makes predictions will enhance trust.
Creating user-friendly interfaces that enable easy interpretation can boost confidence in AI-based medical decisions.
CONCLUSION
As the use of AI tools in healthcare continues to advance, these recommendations can help organisations tackle implementation challenges. By following these guidelines, healthcare organisations can effectively integrate AI tools, unlocking their potential to enhance healthcare outcomes.
ABOUT THE AUTHORS:
Adrian Yeow is an Associate Professor at the School of Business, Singapore University of Social Sciences. He is also an Associate Editor of Journal of the Association for Information Systems (JAIS) and Area Editor for Clinical Systems and Informatics, Health Systems Journal
Foong Pin Sym is a Senior Research Fellow and Head of Design (TeleHealth Core) at the Saw Swee Hock School of Public Health, National University of Singapore.
The content in this article was adapted and updated with permission from Asian Management Insights, Centre for Management Practice, Singapore Management University. The original article can be found here.